Neurons play a key role in encoding sensory stimuli by selectively modulating their activity level for a subset of all possible inputs. This pattern of modulation is often summarized via a tuning curve. One prominent hypothesis, the efficient coding hypothesis, posits that these tuning curves should be shaped to maximize the ability of neuronal populations to encode natural sensory signals. While the most efficient allocation of tuning curves has been calculated in a variety of ways for individual stimulus features, it remains unclear how applicable existing models of tuning curves are for neurons tuned across multiple stimulus features.
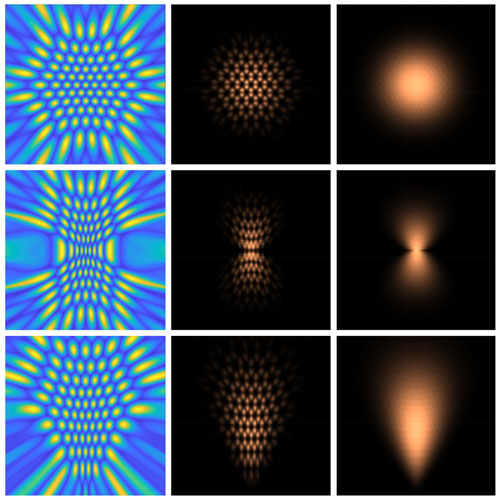
Figure: A visualization of how multidimensional populations of neurons can be warped to encode different features of the natural environment. The panels on the left show simulated populations of neurons, with each neuron represented by a two-dimensional tuning curve. The middle and right panels illustrate the determinant of the Fisher information matrix for sparse and dense neuronal populations. These patterns reveal the underlying stimulus probability distribution that each neuronal population was optimized to encode.
In this report, we made advances towards filling this gap by extending existing frameworks for efficient 1-D population codes to higher dimensions. Similar to prior work in 1-D, the prediction for multi-dimensional populations suggests that regions in stimulus space that are more likely should be represented by a proportionally larger number of neurons, which are each proportionally more selective. The key intuition behind this result is that each neuron in the optimal population is equally likely to fire a spike under natural conditions (that is, when the presented stimuli come from the joint environmental probability distribution of all relevant features).
The import of any theoretical model often hinges on its ability to be verified experimentally. The prediction that neural tuning curves simultaneously encoding multiple dimensions should be distributed proportionally to the joint environmental distribution could be experimentally verified or falsified with a combination of empirical measurements of multidimensional tuning curves and environmental statistics. Interestingly, considering the case of multidimensional stimuli revealed that some efficient coding predictions will likely be more difficult to test experimentally than others. For example, testing some predictions likely requires exhaustive knowledge of all features being coded by the population. Thus, it is important to consider how unmodeled features of biological function (i.e., multidimensional tuning) may manifest themselves during experimental observation.
In addition to these explicit predictions, this work makes it possible to ask questions about the computational benefit of utilizing an optimal multidimensional population as opposed to multiple 1-D populations when performing downstream tasks such as decoding. Critically, our extension to multidimensional heterogenous populations allows for more direct comparisons with other neural coding schemes for higher dimensional signals. The availability of more datasets characterizing neuronal responses across multiple stimulus dimensions should eventually answer the questions of what real neuronal systems are optimized for and what constraints they operate under.